A “granny summary” of an article by Dror Dotan, Ofir Eliahou, and Sharon Cohen
Serial and syntactic processing in the visual analysis of multi-digit numbers
Look at the letter O and the digit 0. See how similar they are. Now look at the letter l (lowercase L) and the digit 1, at S and 5, 9 and g, T and 7 (not identical but still similar), E and 3 (mirrored), G and 6. In fact, this similarity i5 what mak35 it p055ib1e f0r y0u t0 r3ad 3v3n if w3 r3p1ac3 s0m3 l3773r5 with 5imi1ar di9it5 (this writing is known as “leet”, usually spelled “1337”). Still, in spite of this similarity between letters and digits, the cognitive visual mechanisms that recognize letters and digits are separate. They even lie in different brain areas. Strange, isn’t it? I mean, why should the brain “waste” two separate brain regions for handling two tasks which are apparently so similar – namely, recognizing letters and digits? Couldn’t the brain be more efficient, and recognize both letters and digits using a single mechanism?
There are several possible answers to this question. One hypothesis says that to understand this separation, we must transcend the single-character level: although letters and digits are similar, there are critical differences between letter strings and digit strings. The difference is not in their visual properties, but in their structure – i.e., in the rules that dictate which character combinations are valid, and those that dictate which characters are more important than others.
For example, when we write numbers, the digit 0 can’t be the first digit in a string (035 is usually considered as an invalid number). The digit 0 is special for other reasons too: e.g., when you read a number out loud, 0 doesn’t translate into a word. The digit 1 is special too: the decade digit is usually translated into a tens word (2 = twenty, 3 = thirty, etc.), but 1 in the decades gets us a teen word (13 = thirteen). For letter strings, the rules are even more complex. For example, even if you don’t speak English very well, you can be quite sure that “gbncxyq” is not a valid word in English – you see that it doesn’t follow the rules (even if you can’t state them explicitly!). You also probably know that “q” must be followed by “u”, that it is a good idea to treat “th” as a single element rather than as two separate letters, and so on.
Is the visual process of parsing letters and digits indeed sensitive to such special properties? To examine this, we asked whether the visual analysis treats the digits 0 and 1 in a special manner, and precisely how. Our experiment was very simple: the participants saw multi-digit numbers with 3-5 digits, which were presented on the screen for brief durations (≤ 200 milliseconds), and read them aloud (e.g., you see 203 and say “two hundred and three”). We analysed the errors they made: failing to identify a digit (e.g., read 25 as twenty six) or identifying a digit in an incorrect position (e.g., read 25 as fifty two).
The results were very interesting: the frequency of position errors for the digits 0 and 1 was about half than for the digits 2-9 (Fig. 1, bottom). So yes, it seems that the “digit visual anlayzer” treats 0 and 1 in a special manner.
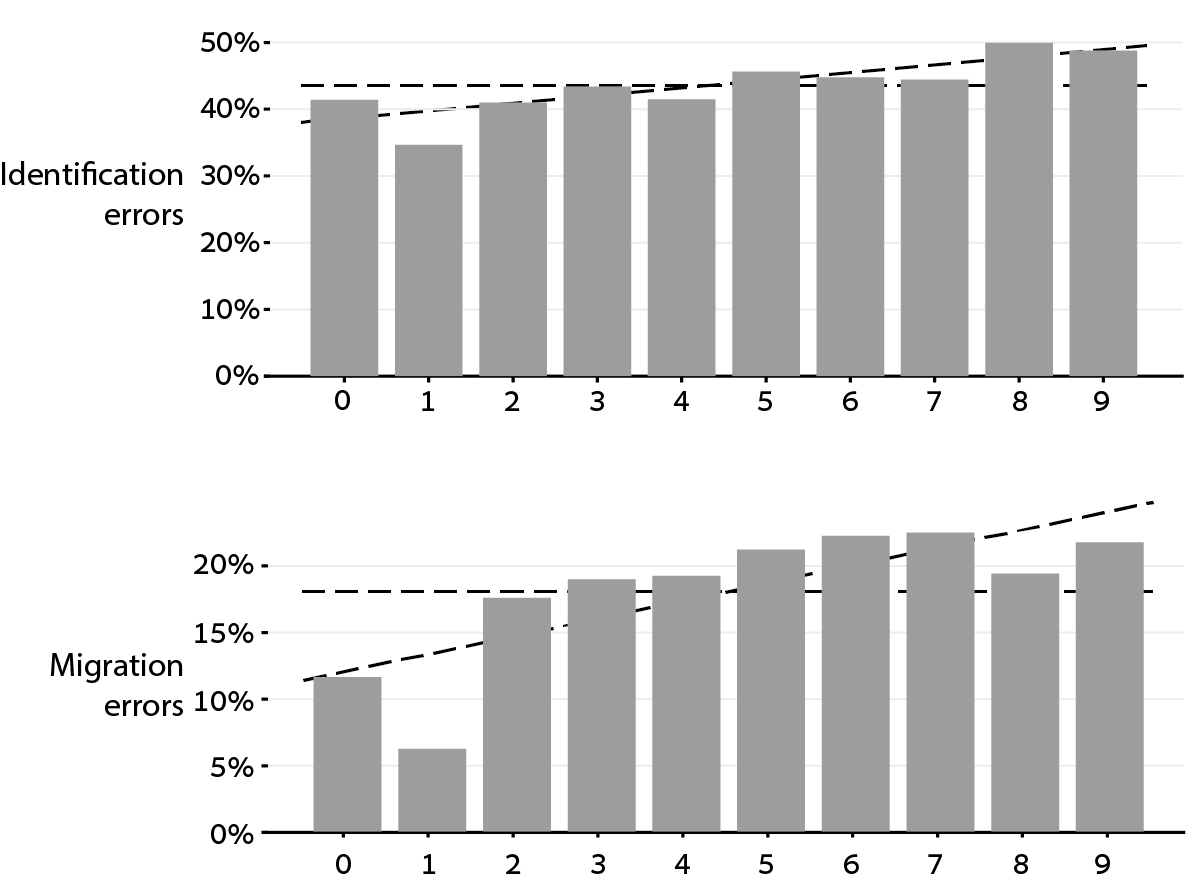
Fig. 1. Identification errors (top) and position errors (bottom) in each digit.
Although the position encoding of 0-1 was superior to that of 2-9, the identity of 0 and 1 was not encoded much better than that of 2-9 (Fig. 1, top). This seemed strange. Why should the visual analyzer have a special treatment for the positions of 0 and 1 but not for their identity?
To explain this, we proposed the following idea. Scientists believe that the digit visual analyzer consists of several “digit detectors”, each of which can detect one digit. You can think of a detector as a machine whose input is the visual information of the relevant digit (e.g., the length and orientation of lines, the position of circles or half-circles), and whose output is the digit identity. We proposed that these detectors are not perfect, in the sense that visual information may “leak” from the input of one detector to the input of an adjacent detector. As a result, the leaking digit may be identified by the adjacent detector too. For example, if the visual information of the digit 3 in the number 234 leaks from the decade detector to the unit detector, the unit detector may think it saw 3, and the person will read the number as 233. To us, this would seem like a position error.
We proposed that to cope with this leakage, the detectors are equipped with an inhibition mechanism: whenever a detector detects a particular digit, it encourages its neighbouring detectors to avoid detecting that digit. In the above example, the decade detector, having detected a “3”, will shout at the unit detector and at the hundred detector, “I don’t know what your digit is, but it’s probably not 3, because 3 is mine”.
How is this related to 0 and 1? We think that because 0 and 1 are particularly important, the brain cares more about getting them in the right position than it cares about other digits. So this inter-detector inhibition mechanism is stronger for 0 and 1 than for other digits.
If this hypothesis is correct, a non-trivial prediction follows: if we analyze the participants’ performance in the digit 2-9 only, we should observe better performance in digits next to 0 or 1 than in digits that are not next to 0 and 1. The reason is that the strong inhibition from the neighbouring 0/1 may help the detector. For example, in the number 302, the inhibition from the decade digit 0 would make it easier to identify the 3 and the 2. Indeed, this is precisely what happens! The probability to make an error in a digit next to 0 or 1 was significantly lower than to make errors in other digits.
Thus, it seems that the digit visual analyzer is adapted to the particularities of 0 and 1. Moreover, these particularities are not handled by some “external” mechanism that operates on top of the digit detectors; rather, they seem to be integrated very deeply into the digit-detection mechanism, as an inter-detector inhibition mechanism. Given this complexity, we may begin to understand why the brain prefers having a dedicated neural network to handle digit strings, and leave the handling of letter strings to other neurons.
Interested in more details? The full article is here.